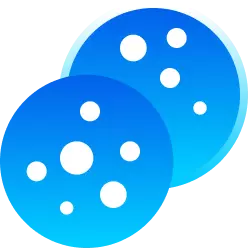
We Use Cookies!!!
We use cookies to ensure that we give you the best experience on our website. Read cookies policies.
In today's interconnected world, migration and emigration have become significant drivers of cultural exchange and diversity. As people move across borders, they bring with them not only their traditions and experiences but also their languages and accents. This phenomenon has led to the emergence of mixed speech accents, which blend linguistic elements from different regions. While these accents enrich our global tapestry, they also pose challenges for technology, particularly Automatic Speech Recognition (ASR) systems. To understand the challenges mixed accents pose, let’s first discuss what speech recognition is.
Speech recognition, or Automatic Speech Recognition, is a technology that converts spoken language into text. It uses neural networks trained on diverse datasets containing audio recordings and corresponding transcriptions. During training, the model learns patterns in acoustic features and text to make accurate transcriptions. ASR is the foundation of voice assistants, transcription services, and more, and its training involves data collection, annotation, model selection, and fine-tuning. ASR technology continually evolves to improve its accuracy and adaptability to various accents and languages, enabling seamless communication between humans and machines.
A speech recognition model needs to understand various accents to convert audio into text. People who migrated from one country to another generally have mixed accents and are often identified by their accents.
To tackle these mixed accent issues, we have to focus on data diversity. So, let’s understand the challenges mixed accents can pose and then see how data diversity can help us improve results.
ASR systems play a pivotal role in transcribing spoken language into written text, powering applications like transcription services, voice assistants, and more. However, the intricate nature of mixed speech accents can prove challenging for these systems. Accents that result from migration can be unique and may not conform to traditional linguistic norms, making them harder to understand for ASR models trained on standard accents.
Mixed speech accents, resulting from migration and cultural exchange, present several notable challenges:
A person migrated to US from India living in US from last 3 years having a mixed accent. If she or he uses the ASR model, then the ASR systems may struggle to accurately transcribe mixed speech accents. The blending of linguistic elements from different regions can make it difficult for models to decipher spoken words correctly, leading to transcription errors.
As I mentioned earlier, we can solve these challenges by including multiple accents in training data. However, collecting and annotating such data can be challenging, as these accents often have unique linguistic characteristics.
ASR models trained predominantly on data from certain regions or accents may exhibit bias against less-represented accents, including mixed speech accents. This bias can lead to unequal recognition performance across different linguistic backgrounds.
Misinterpretations of mixed speech accents can result in frustration and miscommunication, particularly in applications like voice assistants. Users may find it challenging to interact effectively with technology that doesn't understand their speech accurately.
Failing to address the challenges of mixed speech accents can perpetuate inequities and exclude individuals from diverse linguistic backgrounds from the benefits of technology, limiting their access and participation.
Ensuring that ASR systems handle mixed speech accents appropriately is not only a technical concern but also an ethical one. Technology should respect linguistic diversity and promote fair and inclusive communication.
Addressing these challenges involves improving data diversity, enhancing model training techniques, and fostering collaboration between linguists, researchers, and technology developers to create ASR systems that better accommodate mixed speech accents and contribute to a more inclusive technological landscape.
Collecting diverse data can really help us tackle mixed accent challenges because diverse collection allows us to represent all stakeholders. Let’s understand what it means to have diverse data!
Diverse data for ASR models refers to a dataset that encompasses a wide array of spoken language examples, particularly in terms of accents, dialects, languages, and speaking styles. In the context of ASR, diversity includes recordings of people from various linguistic backgrounds, regions, and cultures. This variety is crucial for training ASR models to accurately transcribe speech from a multitude of sources, ensuring they can effectively recognize and understand speech patterns that differ in pronunciation, intonation, and linguistic nuances.
Diverse ASR training data helps these models become more adaptable, robust, and inclusive, enabling them to serve a global user base with accuracy and fairness.
But collecting diverse data is a very challenging task. But this can be solved by asking a few things first when you collect data, and a data partner with expertise in such collection can really help prepare a diverse and balanced dataset to tackle multiple accents!
To make sure the accents of different people, we can ask a few things to participants to make sure their accent. I am sharing a few things that we generally used at FutureBeeAI;
We allow participants to self-identify their accent or dialect. During the data collection process, provide participants with options or free-text fields to describe their accent or linguistic background. This self-identification can help categorize the data effectively.
We ask participants about their place of origin or the regions where they have lived or spent significant time. This information can provide valuable insights into the diversity of accents within the dataset.
We inquire about the languages spoken by the participants and their proficiency in each language. Understanding participants' language backgrounds can help identify multilingual individuals and potential code-switching patterns.
We always request that participants read specific sentences or passages that are known to highlight accent differences. These sentences can include phonetically challenging words or phrases that accentuate distinct pronunciation patterns.
One can also encourage participants to engage in recorded conversations or discussions. Natural conversations often bring out more nuanced aspects of accents compared to scripted readings.
Collecting information about participants' age and their exposure to different accents can also help because accents can evolve over time or through exposure to diverse linguistic environments.
After recording, have trained linguists or annotators review and categorize the data based on accent characteristics. This can help ensure that the dataset is properly labeled for training purposes.
Collaborate with diverse communities and organizations to actively engage individuals with unique accents. This can help ensure a more comprehensive representation of accents in the dataset. Our AI community represents more than 50 countries.
Maintain open channels of communication with participants and gather feedback on the data collection process. Participants' insights and suggestions can be valuable for improving accent diversity.
Our project executives are always in touch with recorders, transcribers, and QA team members to get continuous feedback that helps us improve the results. Also, whenever we get any new accent in the collection, we always convey it to our clients, because many times clients are not aware of different accents spoken in a region.
Having multiple accents is an opportunity for us to build AI models that are more inclusive and representative of the communities they are used by. This can be challenging, but it is possible if we follow a proper data collection strategy and consider data diversity to be a key parameter.
Diverse data is the key to addressing the issue of mixed accents caused by migration and emigration. Collecting data from specific groups of people, such as "Gujarati people living in the US for the past 10 years," can help us target different accents. Additionally, asking questions and taking voice samples before collecting the entire dataset can help us build more balanced datasets.