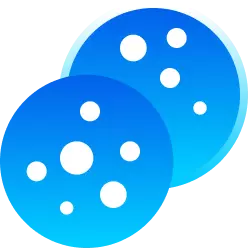
We Use Cookies!!!
We use cookies to ensure that we give you the best experience on our website. Read cookies policies.
In the dynamic world of call centers and automated speech recognition (ASR) systems, high-quality call center speech datasets play a pivotal role. These datasets consist of recorded phone conversations between customers and call center agents, accompanied by accurate transcriptions. They serve as invaluable resources for training and fine-tuning ASR systems, enabling businesses to unlock the full potential of their call center operations.
By harnessing a high-quality call center speech dataset, businesses experience transformative outcomes. The dataset's accurate transcriptions and insights enhance automated speech recognition systems, resulting in improved call center operations, increased customer satisfaction, and personalized responses. By achieving operational excellence, companies create exceptional customer experiences.
To ensure the effectiveness and accuracy of these systems, understanding the key elements that constitute high-quality call center speech data is crucial. This article explores the eight essential elements contributing to the dataset's quality and reliability, empowering businesses to optimize call center operations and elevate customer satisfaction. Utilizing this high-quality call center audio dataset enables building state-of-the-art speech AI solutions for call centers.
When training an industry-specific call center speech recognition model, the audio data and its corresponding transcription serve as the training dataset. It is essential that the audio recordings of agent-customer conversations are clear and intelligible. This ensures accurate transcription and analysis of the speech content.
While it's important to train the ASR model on realistic data that includes background noise or echo encountered in real-life scenarios as an edge use case but having clear and intelligible audio data facilitates transcription and provides accurate ground truths for training the model effectively.
Accurate transcription serves as the foundation for training models effectively. High-quality transcription ensures that audio data is accurately converted into text, providing valuable ground truth for model development. A lower word error rate indicates higher transcription quality.
Precise transcriptions should faithfully represent spoken content, enabling thorough analysis and further processing. Accurate speech-to-text conversion allows for comprehensive conversation analysis, sentiment analysis, and the extraction of call center metrics.
The transcription of call center voice data should involve the following things:
Speaker diversity is a critical aspect of training speech models for call centers. It involves incorporating a wide range of speaker voices to improve the model's accuracy and generalizability in real-life scenarios. There are two key aspects to consider:
To ensure that a call center ASR model performs with equal accuracy for speakers from different regions, states, or provinces, a diverse speech dataset is necessary. This dataset should include speakers from various regions, representing diverse accents and dialects.
By including this diversity, ASR systems can adapt and accurately transcribe speech from different linguistic backgrounds, facilitating a better understanding of customer interactions.
Each speaker has their own unique speech pattern and communication style. Furthermore, different genders may exhibit distinct tonality, voice characteristics, speech patterns, and communication styles.
Therefore, a high-quality call center voice dataset should capture the diverse ways in which people express themselves. This includes variations in speaking rates, pauses, intonations, and emphasis on specific words or phrases.
Training models on such data with a wide range of speech patterns enhance the adaptability and robustness of ASR systems, ensuring accurate recognition of different speech patterns encountered during call center conversations.
Call center conversations encompass a spectrum of speech styles, from formal interactions between agents and customers to more casual exchanges. It is important to note that while agents generally speak in a formal manner, customers may vary in their speech patterns, using both formal and informal language.
For a high-quality call center speech dataset, it is essential to include examples of both formal and informal speech. This allows automated speech recognition (ASR) models to adapt to different communication styles and accurately transcribe the content, capturing the nuances of customer-agent interactions.
In natural speech conversations, interjections, fillers, and pauses are common occurrences. These elements contribute to the authenticity of the call center speech data and help ASR systems handle interruptions and non-verbal cues effectively. Recognizing and accounting for interjections and fillers improves call categorization, sentiment analysis, and overall accuracy in speech transcription.
In a call center setting, various types of interactions occur, including inbound and outbound calls. Inbound calls involve customers reaching out to seek information, resolve issues, file complaints, or provide feedback. On the other hand, outbound calls entail agents contacting customers for information verification, cross-selling, up-selling, or promotional purposes.
When the objective is to develop a speech AI model capable of handling customer calls, transcribing them, extracting insights, and offering suggestions, it is advisable to train the model using inbound call center speech datasets.
Conversely, if the goal is to create AI technology for automating debt collection, sharing information, or conducting promotional campaigns, the speech model should be trained on outbound call center speech data.
Diving deeper, it is crucial to train our conversational AI model on conversations with diverse intents and topics. For example, if we aim to build a speech recognition and conversational AI model specifically for the banking domain to assist with inbound queries, it should be trained on diverse conversations covering various topics, such as:
Additionally, call centers often utilize industry-specific terminology, acronyms, and jargon. Therefore, a comprehensive call center speech dataset should encompass conversations that include a wide range of industry-specific vocabulary. This enables ASR models to understand and transcribe industry-specific terms accurately.
The inclusion of such contextual information allows for more nuanced conversation analysis, enabling businesses to gain valuable insights into customer needs, preferences, and overall satisfaction. It ensures that the AI models are trained on relevant and representative data to perform optimally in real-world call center scenarios.
When working with audio data, it's important to understand the technical features that impact its quality and suitability for training machine learning models. Different devices and communication channels generate audio data with varying sample rates and bit depths. For example, a customer calling from a mobile phone may produce audio with a sample rate of 8 kHz, while using a different channel could result in audio signals with a sample rate of 48 kHz.
To ensure optimal accuracy, it's crucial to train machine learning models on speech data that matches the specific sample rate and bit depth of the customer's communication channel. We typically provide call center speech data with sample rates ranging from 8 kHz to 48 kHz to cover a wide range of scenarios.
The sample rate represents the number of audio samples per second, while bit depth refers to the number of bits used to represent each audio sample
Additionally, when training and fine-tuning conversational AI or ASR models, stereo file speech data is required. This means having separate audio channels for both the agent and the customer. Depending on the use case you're building for, choosing the appropriate file type (stereo or mono) with the correct bit depth and sample rate becomes essential in building a robust speech AI model.
When utilizing a large language-specific call center speech dataset, metadata plays a vital role in visualization, ensuring diversity, emphasizing niche training modes, and facilitating informed decision-making.
It is important to include the following metadata for each audio file in the dataset:
Last but not least. Data privacy and anonymization are critical considerations when acquiring speech datasets for call center automated speech recognition (ASR). Real call center conversations often include personal or sensitive information shared by callers, such as names, addresses, and phone numbers, which could compromise privacy.
To address this concern, it is essential to implement anonymization techniques, removing personally identifiable information (PII) from voice recordings and transcriptions. By doing so, businesses can ensure data privacy and compliance with relevant regulations.
Proper anonymization safeguards customer privacy and ensures the confidentiality of call center speech data. This added layer of protection also allows for secure data sharing and research. Prioritizing data privacy and anonymization contributes to the ethical and responsible use of call center speech data.
Acquiring high-quality, industry-specific call center speech datasets in a specific language with diverse accents, dialects, speakers, and topics can be a challenging endeavor. However, at FutureBeeAI, we simplify this process for you. With our extensive off-the-shelf call center speech dataset available in over 40 languages across various industries, you can seamlessly scale your machine-learning projects.
If you have unique requirements, we can also collect tailored speech data in any language and industry. Our state-of-the-art speech data collection tool and transcription tool, combined with our data expertise and global crowd community, ensure efficient and accurate custom data collection and transcription.
Partner with us to accelerate your call center speech initiative! Let's discuss more about it!