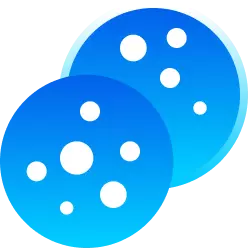
We Use Cookies!!!
We use cookies to ensure that we give you the best experience on our website. Read cookies policies.
The demand for off-the-shelf or ready-to-use speech data is increasing day by day, and after the launch of GEN AI models, people now don’t want to use their time to prepare or custom collect speech data. They just want data that is available and ready for their use case. Earlier, the market size is expected to show an annual growth rate (CAGR 2023-2030) of 16.30%, resulting in a market volume of US$19.57bn by 2030. But now we can see a sudden increase in demand for the speech data. So, I think reaching US$19.57bn will not take 2030.
But with this demand, where people just want to save their time and money, many people are buying poor quality speech data, and poor quality data can lead to a poor model with a loss of time, money, efforts, enthusiasm, market value, and most importantly, your clients.
So, in this blog, I will share some things that you should check and consider while buying any OTS speech datasets. Let’s understand the OTS speech dataset first.
In simple words, an OTS, or ready-to-use speech dataset, refers to a pre-existing collection of audio recordings, often paired with transcriptions or annotations, that are readily available for use in speech-related tasks such as speech recognition, speaker identification, emotion recognition, language modeling, and more.
Using off-the-shelf speech datasets can save time and resources, allowing researchers and developers to focus on building and improving algorithms rather than collecting and processing large amounts of data. However, it's important to carefully choose a dataset that aligns with your specific goals and requirements for the task.
But having a huge amount of OTS data is not enough for developing speech based AI models. Speech data should have some traits in it to help us build better speech recognition models. Let’s discuss some of them.
Speech data has some formats, sample rates, bit rates, and other user related characteristics that make it useful for different use cases. Quality OTS speech data for training models or conducting research typically possesses several key traits:
A diverse dataset includes variability in speakers, accents, ages, genders, languages, and recording conditions. This diversity ensures that the trained models generalize well across different scenarios and populations. For example, if you are building a model that can understand different English accents, then having off-the-shelf data in the US, UK, Indian, Australian, and many other English accents can be helpful if recorded with the help of different age groups and speakers in different environments.
Having accurate and comprehensive annotations or labels is crucial for any quality speech data. They might include manual transcriptions, speaker identities, timestamps, emotions, or any other relevant information for the intended task.
Speech data with proper metadata of speaker age, gender, environment condition, and device specification is very helpful for speech recognition model training.
A well-balanced dataset represents different classes or categories fairly. For instance, in speaker recognition, having an equal distribution of speakers across different demographics ensures unbiased training
Speech data should have a format in which it has been recorded. For different use cases, we mostly need lossless formar wav. Sample rate in speech is also very important. So, having data with a proper sample rate is essential.
OTS data should have proper consent from speakers to avoid ethical issues. Data collection should comply with privacy laws and guidelines, especially when dealing with sensitive information.
The dataset should ideally represent the real-world scenarios or applications for which the model will be used. For instance, if the goal is to create a speech recognition system for a particular region, the dataset should contain speech samples from that region. A combination of these traits contributes to the effectiveness of speech data in training robust and accurate models. So, having these traits is very important for speech data, and you can verify a few things by just looking at the speech data information, but apart from that, you should have a basic understanding of how to check the quality of speech data to avoid breech of traits. Let me share our recent experiment.
We at FutureBeeAI provide better data solutions to build AI models. We provide ready to use datasets as well as custom datasets. With the rise in demand for OTS datasets, we got many requests from our clients for OTS speech data. At the same time, we got many offers from different vendors saying that they wanted to sell their OTS speech data. So, in some cases where we don’t have enough speech data, we asked these vendors to share their data samples. When we checked these samples initially just with a look, they looked pretty fine, and they have already sold this data to many developers. But when we put these data samples into our QA process, we found many quality issues, and these issues can really harm your model training.
Let’s see step by step what we have checked;
The "frame drop issue" in speech data refers to a situation where certain frames or segments of audio data are missing or not properly captured during the recording process.
Each frame represents a small slice of the audio signal, usually a fraction of a second, and missing frames can lead to gaps or inconsistencies in the recorded speech data.
Frame drops in speech data can significantly impact the quality and integrity of the data, potentially leading to inaccuracies or disruptions in tasks such as speech recognition, where continuity and completeness of the audio signal are crucial. Here, you can see what you cannot hear.
A stereo file is an audio file that contains two distinct channels of audio, typically referred to as the left and right channels. The stereo format provides a richer and more nuanced audio experience compared to monaural (mono) recordings, which use a single audio channel.
We got some stereo samples, and we have seen that they are just two monos that combine, where the mono channel contains both speakers voices together. Here you can see the difference;
A) Two mono files are combined and both files have both voices.
B) Correct stereo file, where you can see two different voices in audio waves
Sample rate in audio refers to the number of samples (or measurements) of audio captured per second during the analog-to-digital conversion process. It's measured in hertz (Hz).
In simple words, when sound is recorded digitally, it's sampled at regular intervals, and the sample rate determines how often these samples are taken. A higher sample rate means more samples are taken per second, resulting in a more accurate representation of the original analog sound wave.
Now, for different use cases like speech-to-text for call centers and other general voice use cases, we need 8 or 16 kHz of sample rate, and for Text-to-speech we generally need a 48 kHz sample. The choice of sample rate can significantly impact the quality and characteristics of the recorded audio, and aligning it with the intended use case helps optimize performance and efficiency.
But what if you get 8 kHz sample audio converted to 48 kHz? Or how should you check this? By just looking at audio information, you can never get any idea about the upsampling of audio. And this upsampling will stretch the existing audio data to fit the higher sample rate, resulting in a larger file with no actual increase in audio fidelity. Upsampling will also introduce artifacts or distortions, especially when upsampling significantly (like from 8 kHz to 48 kHz), potentially affecting the sound negatively.
Here you can see the difference and identify this issue;
Here in the image, you can see that the upper portion A has energy for up to 4K and then there is no energy available, which means this audio has been converted from a low sample rate of 8 kHz to 16 kHz, which only increases the size of the audio and not any information. Similarly, you can compare portion B with A, where you see an audio with an 8 kHz sample rate has energy up to 4K, which is correct.
These are the three main issues we found in OTS data samples collected from 11 different vendors across the globe. The total number of samples is 60. These issues can really impact your model training. So, please take care before you buy any OTS speech datasets.
We have developed a data store that has many OTS datasets available and can be helpful in many use cases as it has all the required traits, from diversity to metadata. Also, you will never see any issues like frame drop or sample rate issues, and we can provide stereo, mono, and separate audio recordings.
We have built a robust platform for collecting speech datasets, and with our well trained community, you can also choose a custom collection option for your use cases. Whereas you spend approximately 2 to 5 weeks buying OTS data with some uncertainty in quality, we can deliver up to 1000 hours of data in 8 weeks with transcription in any language.
You can also use our platforms and crowd to review your datasets and get real time updates. So, get in touch if you want to discuss your OTS or custom data needs, or just share a sample of your data and we will check for you!