How to create a voice dataset?
Data collection
Data Annotation
Data splitting
Preparing voice datset for speech recognition involves collection, annotation, cleaning, feature extraction, splitting, and preprocessing to ensure the model learns effectively from diverse and representative samples.
Data Collection: Gather diverse audio samples representing various speakers, accents, and environmental conditions, ensuring coverage of different languages and speech styles.
Data Annotation: Transcribe audio recordings into text, annotating timestamps, speaker information, and metadata like background noise levels and recording quality.
Data Cleaning: Remove irrelevant segments like silence or background noise, normalize audio for consistent volume levels, and eliminate distortions or artifacts.
Feature Extraction: Convert audio signals into numerical representations using techniques like MFCCs or spectrograms, extracting relevant features such as phonemes or words.
Data Splitting: Divide the dataset into training, validation, and test sets while preserving the distribution of speakers and languages.
Preprocessing: Apply normalization, filtering, and resampling to preprocess audio data, along with data augmentation and feature extraction to enhance model robustness and generalization.
What Else Do People Ask?
Related AI Articles
Browse Matching Datasets
Acquiring high-quality AI datasets has never been easier!!!
Get in touch with our AI data expert now!
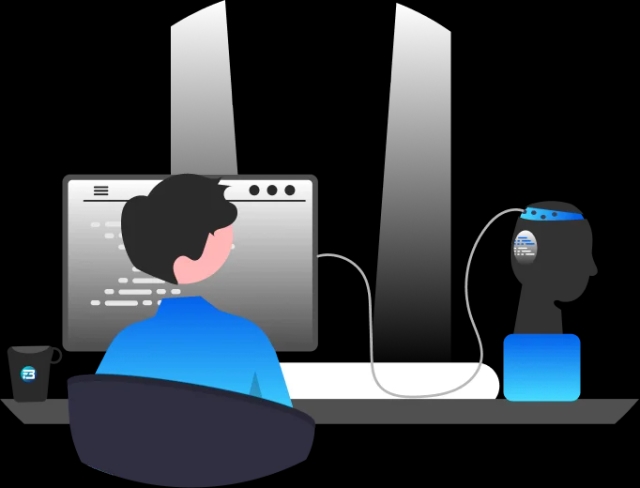