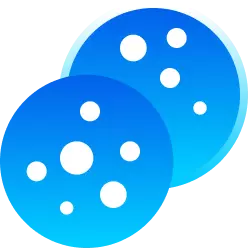
We Use Cookies!!!
We use cookies to ensure that we give you the best experience on our website. Read cookies policies.
Expanding a business is the dream of every business, and effective marketing stands out as a key parameter for achieving this goal by making people aware of the business. Similarly, entertainment and media houses aspire to have their content viewed by a global audience. However, reaching people worldwide is a complex task due to demographic variations, language differences, and evolving customer behaviors. To address these challenges, businesses need to create localized content tailored to each demographic in order to successfully penetrate the market.
Traditionally, companies used human resources to produce multilingual content, but with the recent advancements in artificial intelligence, machine learning models have emerged as a powerful tool for generating multilingual content in both text and voice formats.
One technique that proves valuable in this context is neural machine translation (NMT). However, it's important to recognize that training AI models is a prerequisite for any desired task. When it comes to NMT engines, the training process relies on parallel corpora.
In this blog, we will delve into the concepts of neural machine translation, parallel corpora, and the challenges associated with preparing such corpora. So, let's dive in!
A neural machine translation engine is a type of AI model designed to automatically translate text or speech from one language to another. It utilizes neural networks, which are computational models inspired by the structure and function of the human brain. The NMT engine is trained on parallel corpora, which consist of pairs of sentences in different languages, allowing the model to learn the patterns and relationships between them.
In simpler terms, an NMT engine is like a smart language translator powered by AI. It can understand and generate translations between languages, making it a valuable tool for businesses, content creators, and anyone seeking to bridge language barriers in communication.
With recent advancements, the demand for these types of models has increased, as has the demand for parallel corpora. Like other AI models, parallel corpora are the lifeblood of any NMT engine. So, let's understand parallel corpora.
When we talk about "parallel corpora for machine translation" or "training data for the MT engine," we mean having sets of sentences in multiple languages that are translations of each other.
"Parallel corpora are collections of translations, typically in two languages, that are aligned at the sentence or phrase level.тАЭ
Imagine you have two books, one in English and the other in Hindi. Each page in the English book has a corresponding page in Hindi with the same meaning. This pair of books is like a parallel corpus.
See the table below for a better understanding. English sentences are translated into Hindi to prepare training data for a machine translation model; each pair of English and Hindi is considered a parallel corpus.
English | Hindi |
---|---|
Virat Kohli will make a comeback, right? Team India seems incomplete without him! | рд╡рд┐рд░рд╛рдЯ рдХреЛрд╣рд▓реА рд╡рд╛рдкрд╕реА рдХрд░реЗрдЧрд╛ рдирд╛? рдЯреАрдо рдЗрдВрдбрд┐рдпрд╛ рдмрд┐рдирд╛ рдЙрд╕рдХреЗ рдЕрдзреВрд░реА рд▓рдЧрддреА рд╣реИ!" |
It was an exciting match against New Zealand, there was tension till the end! | рдиреНрдпреВрдЬрд╝реАрд▓реИрдВрдб рдХреЗ рдЦрд┐рд▓рд╛рдл рддреЛ рд░реЛрдорд╛рдВрдЪрдХ рдореИрдЪ рдерд╛, рдЖрдЦрд┐рд░ рддрдХ рдЯреЗрдВрд╢рди рдерд╛! |
What amazing films are being made in the Telugu industry! | рддреЗрд▓реБрдЧреВ рдЗрдВрдбрд╕реНрдЯреНрд░реА рдореЗрдВ рдХреНрдпрд╛ рдХрдорд╛рд▓ рдХреА рдлрд┐рд▓реНрдореЗрдВ рдмрди рд░рд╣реА рд╣реИрдВ! |
Hey man, I feel like watching AR Rahman's live concert! | рдЕрд░реЗ рдпрд╛рд░, рдореЗрд░рд╛ рддреЛ рдорди рдХрд░рддрд╛ рд╣реИ рдП рдЖрд░ рд░рд╣рдорд╛рди рдХрд╛ рд▓рд╛рдЗрд╡ рдХрдВрд╕рд░реНрдЯ рджреЗрдЦреВрдВ! |
Nowdays, Bollywood songs have become completely useless, not as fun as in the old times! | рдЖрдЬрдХрд▓ рдмреЙрд▓реАрд╡реБрдб рд╕реЙрдиреНрдЧреНрд╕ рдмрд┐рд▓рдХреБрд▓ рдмреЗрдХрд╛рд░ рд╣реЛ рдЧрдП рд╣реИрдВ, рдкреБрд░рд╛рдиреЗ рдЬрд╝рдорд╛рдиреЗ рд╕рд╛ рдордЬрд╝рд╛ рдирд╣реАрдВ! |
Hrithik Roshan is my favorite actor, no one has style like him! | рдЛрддрд┐рдХ рд░реЛрд╢рди рддреЛ рдореЗрд░рд╛ рдлреЗрд╡рд░реЗрдЯ рдПрдХреНрдЯрд░ рд╣реИ, рдЙрд╕рдХреЗ рдЬреИрд╕рд╛ рд╕реНрдЯрд╛рдЗрд▓ рдХрд┐рд╕реА рдХрд╛ рдирд╣реАрдВ! |
A parallel corpus is a key element of the MT Engine; it can help train, test, and fine tune any translation specific AI model. But preparing a parallel corpus is not an easy task and requires expert human force to build high quality data.
Parallel corpora can be prepared for text-to-text and voice-to-voice. In voice to voice, we have to record the same sentences in different languages with the same recorders, also known as speech parallel corpora.
Moving forward, we will discuss the challenges of preparing parallel corpora.
Creating parallel corpora, which are sets of texts in one language aligned with their translations in another language, poses several challenges. Here are some common challenges faced in preparing parallel corpora:
You may have heard garbage in garbage out, which means the quality of the output is determined by the quality of the input. So, to produce quality translations with the help of AI, we need to feed high quality parallel corpora and ensuring accurate and high-quality translations is a significant challenge. Translations need to be faithful to the source text, capturing both the literal meaning and the nuances.
Maintaining consistency in translation style and terminology across different translators or translation projects can be challenging. Inconsistencies can hinder the effectiveness of the parallel corpus. Also, languages can have different grammatical structures, word orders, and idiomatic expressions. Aligning these structures accurately in a parallel corpus can be complex.
If the content of the parallel corpus is domain-specific, finding translators who are proficient in both the source and target domains can be challenging. Specialized terminology may not have direct equivalents in the target language.
Languages often have multiple dialects, and finding appropriate parallel data for each dialect can be challenging. This is particularly important for languages with significant regional variations.
Obtaining permission to use and distribute translations, especially if they are not original works but adaptations, can pose legal challenges. Copyright considerations are crucial when compiling and sharing parallel corpora.
These are some of the main challenges that you can face while creating parallel corpora. We cannot compromise on the quality, consistency, domain expertise, or ethical collection of such data. In most cases, we have to prepare the data because the available open source data is not enough and one cannot use it for commercial purposes for many reasons.
To overcome all these challenges, FutureBeeAI can be your data collection and preparation partner.
We offer custom parallel corpora data collection services as well as ready to use datasets in more than 50 languages. We have built some SOTA platforms that can be used to prepare the data with the help of our crowd community in multiple languages.
Our data can save you from copyright and legal issues and allow you to get domain specific multiple dialect data.
You can contact us for samples and platform reviews.