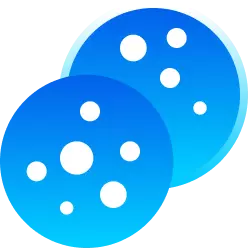
We Use Cookies!!!
We use cookies to ensure that we give you the best experience on our website. Read cookies policies.
Computers have become super smart at reading text from pictures and videos, thanks to optical character recognition (OCR). This technology has changed how we handle documents, making things way more accurate and faster, especially in fields like finance, retail, healthcare, and education.
OCR traces its roots back to telegraphy. On the eve of the First World War, physicist Emanuel Goldberg invented a machine that could read characters and convert them into telegraph code. In the 1920s, he went a step further and created the first electronic document retrieval system.
At this time, businesses were microfilming financial records—great in principle, but quickly retrieving specific records from spools of film was nearly impossible. To overcome this, Goldberg used a photoelectric cell to do pattern recognition with the help of a movie projector. By repurposing existing technologies, he took the first steps toward the automation of record keeping.
Today, OCR can handle lots of different fonts and even handwriting or not-so-clear pictures, though sometimes it might not be perfect, especially with tricky fonts or blurry text.
Then comes AI. The AI-powered OCR uses machine learning algorithms that can handle complex handwriting and blurry pictures.
In this blog, we will discuss what OCR is and the application of AI-powered OCR.
The terms text recognition and optical character recognition are often used interchangeably. Text recognition is used to extract the given text from an image or document. Or we can say that OCR or text recognition, is a process that converts text from images to a machine-readable text format so that it can be modified and stored digitally.
With the development of AI technology, we now use AI-powered OCR tools to extract and read text from documents. So, let’s discuss different OCR systems with or without AI.
A simple OCR engine works by storing many different font and text image patterns as templates. The OCR software uses pattern-matching algorithms to compare text images, character by character, to its internal database. If the system matches the text word for word, it is called optical word recognition.
This solution has limitations because there are virtually unlimited font and handwriting styles, and every single type cannot be captured and stored in the database.
Modern OCR systems use intelligent character recognition (ICR) technology to read the text in the same way humans do. They use advanced methods that train machines to behave like humans by using machine learning algorithms. A machine learning system called a neural network analyzes the text over many levels, processing the image repeatedly. It looks for different image attributes, such as curves, lines, intersections, and loops, and combines the results of all these different levels of analysis to get the final result. This process includes computer vision for text recognition.
This type of system needs a lot of training data to get trained but it provides better and quicker results compared to a simple OCR system. Here, the challenge is to collect high quality image-text data because the accuracy and effectiveness of OCR heavily rely on the quality, diversity, and representativeness of the training data. Biased or incomplete datasets can impact performance.
To understand the impact of OCR or text recognition, we have to understand its importance.
In most businesses, they get a lot of information on paper—like forms, invoices, or contracts. Dealing with all that paper takes up tons of space and time. But going paperless is the way to go! The tricky part is when you scan these documents into a computer. They become pictures, not text, making it hard for computers to read and use the words.
Here's where OCR helps out! It turns those picture words into actual text that computers can understand. Then, you can use that text for all sorts of things—like figuring out patterns, making work easier, or even automating tasks. Basically, OCR turns picture words into useful computer data that helps businesses work smarter and faster.
Digitalization is a way forward and the future of OCR is bright!
Most businesses use OCR or text recognition, systems for many purposes. Let's discuss some of them;
OCR helps in swiftly processing checks, invoices, and various financial forms, reducing the need for manual data entry.
By scanning and analyzing documents, OCR aids in identifying inconsistencies or potential fraudulent activities within financial records.
OCR converts handwritten medical records into digital formats, making them easily accessible and allowing for quick analysis and retrieval.
It assists in transcribing doctors handwritten notes or prescriptions into digital text for better management and analysis.
OCR reads barcodes or labels, facilitating efficient tracking and management of inventory.
Optical character recognition helps in extracting information from customer forms, enabling personalized services and quicker response times.
OCR processes legal documents for review, searching, or extracting specific information required for legal proceedings.
It aids in digitizing contracts and managing large volumes of legal paperwork, improving accessibility and organization.
OCR automates grading processes for exams or assignments, saving time for educators and providing quicker feedback to students.
It assists in converting textbooks or printed materials into digital formats, expanding accessibility and ease of distribution.
OCR reads shipping labels and documents, streamlining logistics operations and ensuring accurate tracking of goods.
It aids in reading product codes or labels, ensuring adherence to quality standards during production and distribution processes.
OCR digitizes historical documents or archives for preservation and easier accessibility, aiding in efficient administration.
It assists in processing and verifying identity documents swiftly and accurately for various administrative purposes.
OCR expedites the processing of insurance claims by extracting relevant information from documents, reducing processing times.
It automates data extraction and analysis for policy-related documents, improving efficiency in managing insurance policies.
These are some of the examples where we can see the uses of OCR technology.
Training a text recognition model requires a comprehensive and diverse dataset, appropriate architecture selection, effective training strategies, and continuous improvement to achieve robust and accurate recognition. Let’s discuss some of the key steps that you should follow;
Gathering a diverse dataset containing text samples from a language you intend to support. Include a wide range of fonts, styles, and contexts to make the model robust. Depending on your use case, you can collect images of storefronts, books, invoices, bank statements, magazines, etc.
Before feeding the images into the model, they must be preprocessed to improve their quality and make them easier to analyze. This might involve cropping the images, enhancing the contrast, and removing any noise or distortion.
Once we collect the data, we have to transcribe all the text from the images so that the model can learn to detect and recognize pixel patterns as characters.
Train the model using the annotated dataset. And if you are building a multilingual model, then implement techniques like transfer learning, where the model learns patterns from one language and adapts to others, leveraging shared characteristics between languages.
Fine-tune the model on multilingual datasets, adjusting parameters to enhance its performance across various languages. Evaluate the model’s accuracy, precision, and recall for each supported language separately to ensure balanced performance.
We offer custom image data collection services as well as ready to use datasets in more than 50 languages. We have built some SOTA platforms that can be used to transcribe text from images with the help of our crowd community in multiple languages. You can contact us for samples and platform reviews.