Generative AI is a rapidly growing field with many potential applications. It has the potential to revolutionize many industries, including healthcare, entertainment, and education. We are still in the early days of generative AI, but it is clear that this technology has the potential to change the world.
One of the most exciting applications of generative AI is the creation of new content. These models can be used to create realistic images, videos, and even music. This has the potential to revolutionize the entertainment industry, as well as the way we consume news and information.
Here are a few more examples to understand how powerful Generative AI is: Generative AI can also be used to create new educational materials. For example, generative AI models can be used to create personalized learning experiences or to generate interactive simulations. This has the potential to make education more engaging and effective.
In the healthcare industry, generative AI can be used to create new drugs and treatments. For example, it can be used to design new molecules or to simulate the effects of drugs on the human body. This has the potential to revolutionize the way we treat diseases.
Generative AI is a powerful technology with many potential applications. However, it is also a rapidly growing field, and there are a number of challenges that need to be addressed before generative AI can be widely adopted.
In this blog, we will take a deep dive into the 5 biggest challenges that Generative AI is facing and can become a potential threat to Generative AI as well as human beings.
What is Generative AI?
Generative AI is a type of Artificial intelligence that can generate new content based on the input. Generative models are trained on huge amounts of training data to generate novel content and it can generate all types of media content, it can be audio, text, video, image, and even codes.
It’s so fascinating to see and use generative AI models, and how easily they can produce what you want for some specific use cases. Literally, they can like increase your efficiency by many folds but it needs to be the right person to use these generative models in an ethical way.
I am sure you have heard about OpenAI’s large language model chatGPT 4 which has taken the world by storm, while it has given opportunity improve our creativity, and it has also raised concerns about potential threats.
“Every coin has two sides” means the same as there are two sides to every story, and it expresses a similar sentiment to, “don't judge until you've walked a mile in their shoes”
So, I must say that “don’t trust blindly Generative AI until you know how it can harm you”, it can produce a false statement, and may not have any proper data to answer your queries, it may give you output related to someone specific, and I am sure, you would not like to get a positive opinion on any political party in all conditions, are you?
Generative AI has huge potential to change how we work and how we think about different things. To harness the true potential of Generative AI, we should know the challenges facing Generative AI.
Biggest Challenges Facing Generative AI
Let’s discuss all 5 challenges one by one.
Data Availability and Quality
Data quality and quantity are significant challenges in generative AI. To train generative AI models effectively, large amounts of high-quality data are required. However, obtaining such data can be challenging for several reasons.
Collecting and generating high-quality data can be expensive and time-consuming. For instance, in natural language processing tasks, like text generation, human-generated data with accurate annotations or labels is often needed. This process requires human experts to review and annotate the data, which can be a labor-intensive and costly endeavor.
Some domains may have limited availability of data. In specialized or niche domains, obtaining a substantial amount of relevant and diverse data can be particularly challenging. This limitation can impact the performance and generalization ability of generative AI models, as they may not have enough representative data to learn from.
Ensuring the quality of the data is crucial. Noisy or inaccurate data can have a detrimental effect on the performance of generative AI models. Data might contain errors, biases, or misleading information, which can be unintentionally learned and reflected in the generated outputs. Therefore, data preprocessing and cleaning are essential steps to ensure data quality.
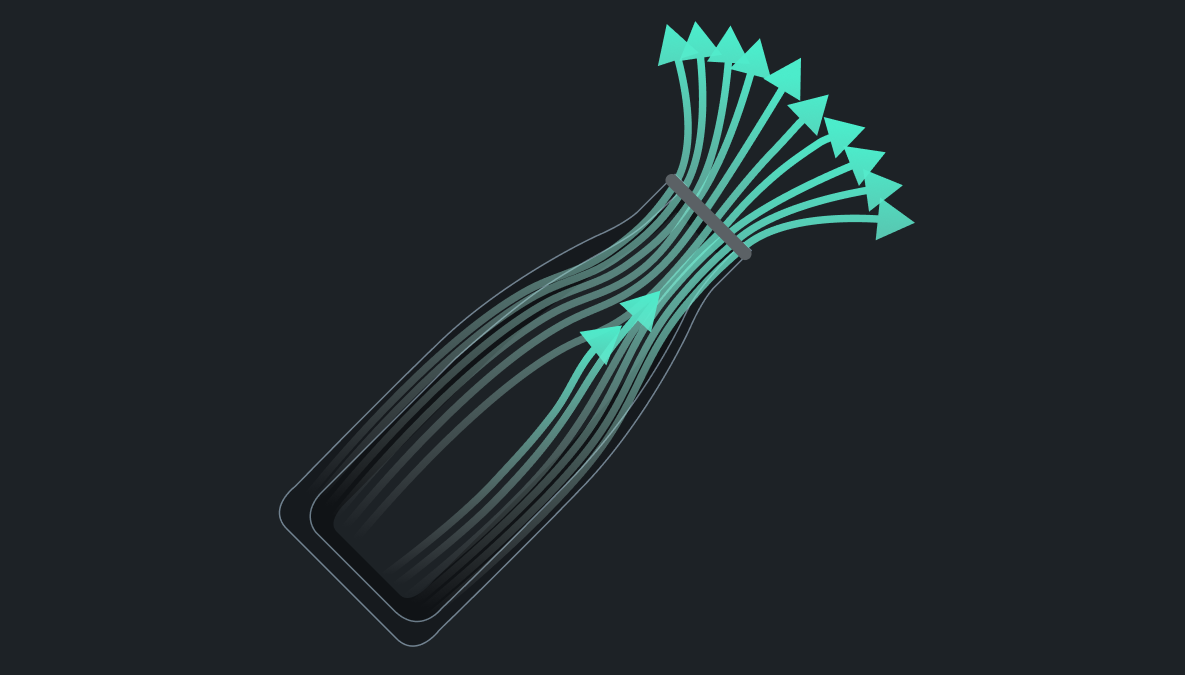
In simple words, data preparation for developing Generative AI is a very challenging process and it may also face the data bottleneck.
Well, in a nutshell, the number of data sets being used by AI models for training has grown in size by around 50 percent each year, while the total stock of language data available to train on is only growing by 7 percent a year, say the researchers, and so won’t be able to keep up with demand.
Bais
Generative AI models can be biased, reflecting the biases that exist in the data they are trained on. This can lead to the creation of content that is inaccurate, harmful, or offensive.
Bias refers to systematic favoritism or prejudice towards certain groups or individuals. In generative AI, bias can arise when the training data used to develop the models contains inherent biases or reflects societal prejudices. For example, if a generative AI model is trained on text data that predominantly includes biased or stereotypical portrayals of certain demographic groups, the generated content may also exhibit those biases.
Bias can manifest in various ways, such as gender bias, racial bias, or bias towards specific professions.
Let’s understand this with another example: A Generative AI that is trained on data that favors a particular political party can lead to a change in the election result in any country and as we know Generative AI needs a lot of funds and richer countries may make small models against some political parties and change the government in poor countries.
So, it’s very crucial to have bias-free training data.
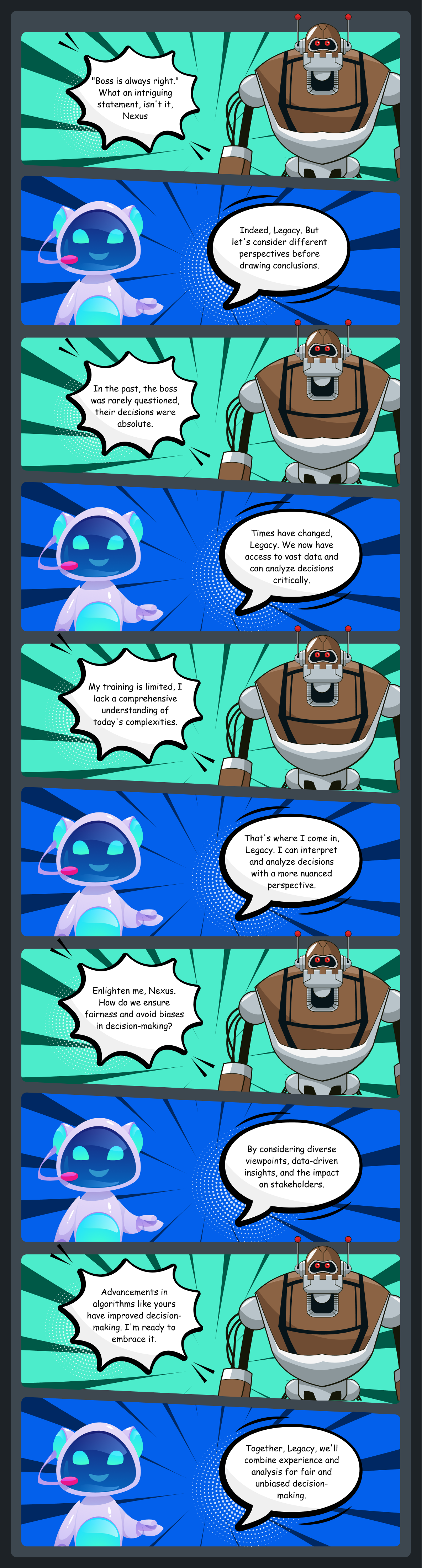
Security
The security challenge in generative AI revolves around preventing malicious use and protecting against harmful consequences.
Generative AI models can be used to create counterfeit content, such as fake news articles or deepfakes. This can have a negative impact on society and the economy.
Here are some of the ways that generative AI can be used to create counterfeit content:
Detecting and mitigating deep fakes, securing generative AI models and data against unauthorized access or tampering, and developing robust defenses against adversarial attacks are key aspects of addressing security challenges.
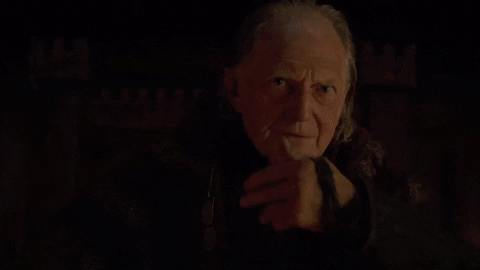
Intellectual Property (IP) Rights
The issue of IP rights in generative AI arises due to the question of ownership and legal protection for AI-generated content. Determining who owns the rights to content created by generative AI models, such as artwork or music, can be complex. This challenge requires legal frameworks and regulations to establish clarity and fairness regarding IP rights in the context of generative AI. It involves considering factors like the contributions of human creators, the role of AI systems, and the potential for collaborations between humans and machines.
Regulation
Generative AI poses regulatory challenges due to its rapid development and potential impact on various aspects of society. The lack of comprehensive regulations specifically tailored to generative AI can lead to misuse, ethical concerns, and unintended consequences. Establishing responsible regulations that balance innovation and societal well-being is crucial. This involves addressing issues like data privacy, fairness, transparency, explainability, and accountability in generative AI systems. It requires collaboration between policymakers, researchers, and industry stakeholders to develop effective regulatory frameworks and ethical guidelines.
Before We Get to the Final Thoughts
Before we conclude this blog, I would like to share one hyped word, Hallucination.
Hallucination refers to the phenomenon where generative AI models produce outputs that do not correspond to reality or the training data. It occurs when the models generate content that goes beyond what they have learned from the training data, resulting in the creation of unrealistic or nonsensical outputs.
If you want to know more about Hallucination, then open Bard or Chatgpt and ask
-What is AI Hallucination?
-Why do you produce
hallucinations?
-How to overcome hallucinations?
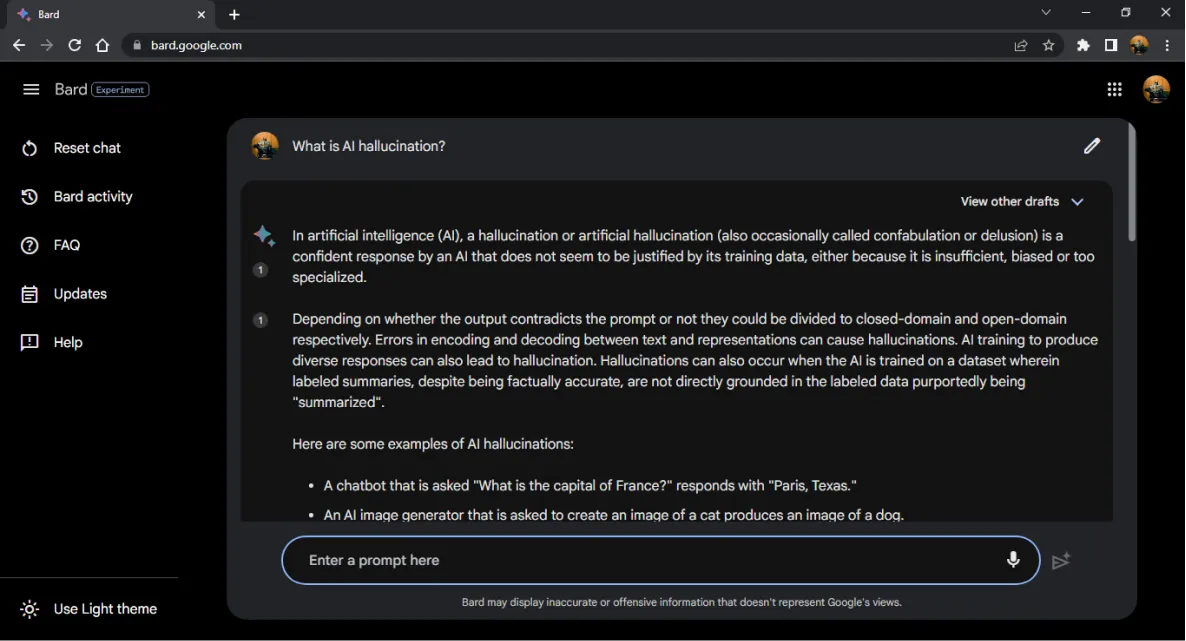
Final Thoughts
Generative AI is a powerful technology with many potential benefits. It has the potential to revolutionize many industries, such as content creation, fraud detection, and drug discovery. However, there are also a number of challenges that need to be addressed before generative AI can be widely adopted. These challenges include data availability and quality, bias, security, intellectual property rights, and interpretability. By addressing these challenges, it is possible to develop and deploy this technology in a way that is beneficial to society.