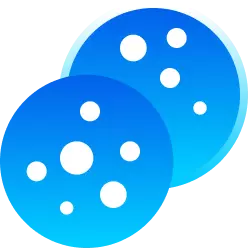
We Use Cookies!!!
We use cookies to ensure that we give you the best experience on our website. Read cookies policies.
Artificial intelligence is making decisions about our lives. Will you get this job, or will you get admission to a particular college? This is also decided by AI in many places. AI systems have become an integral part of our lives. However, the rapid advancement of AI technology comes with a set of ethical and societal challenges, chief among them being the issue of trust. How do we trust AI systems to make unbiased decisions, protect our privacy, and ensure our safety? The answer lies in what I like to call the five pillars of socio-technological responsibility, or the five pillars to building trust in AI systems. So, let’s quickly discuss them one by one.
Fairness is the cornerstone of trust in AI systems. It demands that AI algorithms and models treat all individuals and groups equitably, without any form of bias or discrimination. Achieving fairness in AI is not just a matter of ethics; it's a fundamental requirement to ensure that AI benefits everyone. Fairness can be achieved by training a model on a use case-specific diverse training data andevaluating model outputs from time to time.
Imagine an AI system used in hiring processes that unintentionally favors one demographic over another. Such bias not only harms those discriminated against but also erodes trust in the system. Fairness ensures that AI systems do not perpetuate or amplify existing inequalities.
Let's consider a loan approval AI model used by a financial institution. To ensure fairness, the model should be trained on a diverse dataset that represents applicants from various backgrounds, including different genders, races, and income levels. Regular audits and bias checks should be conducted to identify and rectify any discriminatory patterns. By implementing fairness in this AI system, the aim is to make sure that loans are approved or denied solely based on the applicant's creditworthiness and not influenced by factors unrelated to their ability to repay the loan. This fosters trust in the fairness and integrity of the AI-driven loan approval process.
Explainability is an essential component of building trust in AI systems. It centers on the capacity of AI algorithms to provide clear and understandable explanations for their decisions and predictions. When AI systems can explain their reasoning, it enhances transparency, accountability, and user confidence in their outcomes.
AI models are often complex and function as "black boxes," making it challenging to discern how they reach specific conclusions. This lack of transparency can lead to skepticism and mistrust. Explainability addresses this issue by enabling users to comprehend why an AI system makes a particular decision.
Consider a medical diagnostic AI is used to identify diseases from medical images like X-rays or MRIs. In a healthcare setting, understanding why the AI system made a particular diagnosis is critical for the attending physician and the patient. If the AI system can provide a clear explanation, such as highlighting the regions of an image that contributed to its decision or referencing specific medical guidelines and research, it can instill trust in both the medical professionals and the patients.
For instance, the AI might explain that it detected a certain anomaly in the X-ray, provide references to relevant medical literature, and detail the criteria it used to reach the conclusion. This level of transparency and explainability ensures that the medical team and the patient can trust and rely on the AI's diagnostic recommendations.
Robustness is a fundamental pillar for establishing trust in AI systems. It pertains to the AI's ability to maintain its performance and integrity even when faced with challenges, external threats, or adversarial attempts to manipulate it. A robust AI system can withstand unexpected situations and maintain reliable functionality.
The real world is filled with uncertainties, including security threats, adversarial attacks, and unexpected variations in data. A lack of robustness can expose AI systems to vulnerabilities and erode trust. A robust AI system, on the other hand, ensures that it remains dependable even in adverse conditions.
Imagine an autonomous vehicle equipped with AI for navigation and safety. This vehicle operates in various environments, including unpredictable weather conditions and busy city streets. Robustness is crucial to ensuring that the AI system controlling the vehicle can handle unexpected situations.
For instance, if the vehicle's sensors detect an obstacle on the road due to sudden heavy fog, the AI system needs to respond appropriately by slowing down or making necessary adjustments to maintain safety. Robustness in this context involves the AI's capability to adapt to challenging conditions, ensure the vehicle's safety, and prevent accidents.
Furthermore, robust AI systems are resistant to adversarial attacks. For example, if someone tries to manipulate the vehicle's sensors to mislead the AI, a robust AI should be able to detect such attempts and continue functioning safely. By demonstrating this level of robustness, the AI in autonomous vehicles instills trust in passengers and the general public, making them feel secure when relying on AI-driven transportation solutions.
This is the fourth and pivotal pillar for building trust in AI systems. It involves providing comprehensive information about how AI systems function, their capabilities, and their limitations. Transparent AI systems empower users to make informed decisions and better understand the technology they interact with.
Lack of transparency can lead to distrust and skepticism about AI systems. By making AI transparent, we enhance accountability, facilitate regulatory compliance, and ensure that users know what to expect from these systems.
Let's consider a chatbot used in customer service for an e-commerce platform. Transparency in this context involves several aspects:
The e-commerce company provides fact sheets that summarize the chatbot's purpose, capabilities, and limitations. These documents are easily accessible to customers on the company's website. They include details about what the chatbot can assist with and what it cannot.
Sharing metadata about the data sources used to train the chatbot allows customers to see that the chatbot has been trained on a wide range of customer inquiries and data from product listings and customer reviews.
The company ensures that the chatbot complies with data privacy regulations and has mechanisms in place to safeguard customer data. This compliance is explicitly mentioned in the chatbot's transparency documents.
By providing this transparency, the e-commerce company establishes trust among its customers. They know what to expect from the chatbot, understand its limitations, and have confidence that their data is handled responsibly. This level of openness encourages customers to use the chatbot for support and enhances their overall experience with the platform.
Data privacy is the fifth and most critical pillar for building trust in AI systems. It centers on the responsible handling of personal and sensitive data in AI applications. Privacy ensures that individual’s information is protected and their rights are respected throughout the AI system's lifecycle.
Protecting personal data is both a legal and ethical requirement. Violating privacy can result in severe consequences, erode trust, and damage an organization's reputation. By prioritizing privacy in AI systems, you demonstrate respect for user rights and build trust. A data breach can also cause damage to individuals.
Consider a healthcare AI application used to assist doctors in diagnosing and treating patients. Privacy in this context involves several aspects:
The AI application collects only the data necessary for its intended purpose. It avoids the collection of excessive or irrelevant information, ensuring that it minimizes privacy risks.
Patient data used for training and testing the AI model is anonymized to remove any personally identifiable information (PII). This protects the privacy of patients while still enabling effective AI learning.
All patient data, including medical records and test results, is securely stored and transmitted using encryption. This ensures that the data remains confidential and protected from unauthorized access.
The AI application strictly adheres to healthcare data protection regulations, such as the Health Insurance Portability and Accountability Act (HIPAA) in the United States. Patient’s informed consent is obtained before using their data in the AI system, and compliance with these regulations is thoroughly maintained.
By prioritizing privacy in this healthcare AI application, patients can trust that their personal medical information is handled with the utmost care and respect. This trust is essential for both patients and healthcare professionals who rely on the AI system to enhance diagnosis and treatment decisions while safeguarding sensitive healthcare data.
In this journey towards trustworthy AI, it's essential to understand that we're not just dealing with technology; we're architects of a future where humans and AI coexist harmoniously. Our mission is to create AI systems that align seamlessly with human values and expectations, ensuring they become a force for good in our world.
Trust is the cornerstone of this endeavor. So, as we move forward in this exciting AI revolution, let's remember that building trust in AI is not just a technological challenge; it's a socio-technological imperative. Together, we can shape a future where AI systems enrich our lives while respecting our values and rights.
These five pillars are just a starting point, and as we move forward, we may have to follow more pillars that can shape an AI revolution.
“AI for All with Transparency, Explainability, Robustness, and Privacy"